Prof. Bartz-Beielstein invited to be a keynote speaker at SAEOpt workshop
The SAEOpt workshop to be held at GECCO 2021 in Lille aims to promote the research on surrogate-assisted evolutionary optimisation, particularly the synergies between evolutionary optimisation and machine learning.
GECCO
The Genetic and Evolutionary Computation Conference (GECCO) presents the latest high-quality results in genetic and evolutionary computation since 1999. Topics include: genetic algorithms, genetic programming, ant colony optimization and swarm intelligence, complex systems (artificial life, robotics, evolvable hardware, generative and developmental systems, artificial immune systems), digital entertainment technologies and arts, evolutionary combinatorial optimization and metaheuristics, evolutionary machine learning, evolutionary multiobjective optimization, evolutionary numerical optimization, real world applications, search-based software engineering, theory and more.
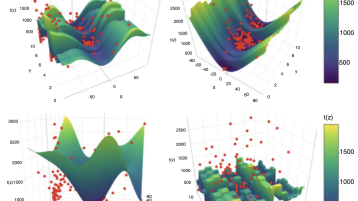
Surrogate-assisted Optimization
In many real world optimisation problems evaluating the objective function(s) is expensive, perhaps requiring days of computation for a single evaluation. Surrogate-assisted optimisation attempts to alleviate this problem by employing computationally cheap 'surrogate' models to estimate the objective function(s) or the ranking relationships of the candidate solutions.
Surrogate-assisted approaches have been widely used across the field of evolutionary optimisation, including continuous and discrete variable problems, although little work has been done on combinatorial problems. Surrogates have been employed in solving a variety of optimisation problems, such as multi-objective optimisation, dynamic optimisation, and robust optimisation. Surrogate-assisted methods have also found successful applications to aerodynamic design optimisation, structural design optimisation, data-driven optimisation, chip design, drug design, robotics and many more. Most interestingly, the need for on-line learning of the surrogates has led to a fruitful crossover between the machine learning and evolutionary optimisation communities, where advanced learning techniques such as ensemble learning, active learning, semi-supervised learning and transfer learning have been employed in surrogate construction.
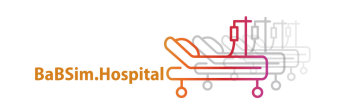
Sequential Parameter Optimization
Sequential Parameter Optimization (SPOT) is an established approach that is based on surrogate models. It has been shown to be one of the most efficient and robost optimization tools. Prof. Bartz-Beielstein and his team have applied SPOT in several real-world optimization problems, e.g., in the BaBSimHospital resource planning task, where a more than 30-dimensional problem is optimized under very limited time constraints.
SAEOpt Workshop
Topics of interest include (but are not limited to):
- Learning approaches for constructing surrogates
- Model management in surrogate-assisted optimisation
- Multi-level, multi-fidelity surrogates
- Complexity and efficiency of surrogate-assisted methods
- Surrogates-assisted evolutionary optimisation of computationally expensive problems
- Data-driven optimisation
- Model approximation in dynamic, robust and multi-modal optimisation
- Model approximation in multi- and many-objective optimisation
- Comparison of different modelling methods in surrogate construction
- Surrogate-assisted identification of the feasible region
- Comparison of evolutionary and non-evolutionary approaches with surrogate models
- Performance assessment and improvement techniques in surrogate-assisted evolutionary computation
Januar 2021