Nominierung für den IEEE CIBCB Best Paper Award
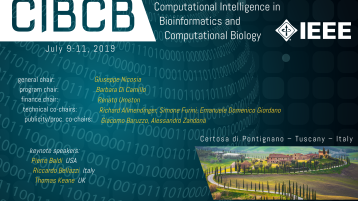
Patrick Echtenbruck ist seit letztem Jahr Promovend am Forschungsinstitut InnovAGe und wird von Prof. Dr. Boris Naujoks am Institut IDE+A betreut. Nun wurde seine Publikation zum Thema "A Multiobjective Approach to Classification in Drug Discovery" bei der 16th CIBCB eingereicht, akzeptiert und für den Best Paper Award der Konferenz nominiert.
Gemeinsam mit Michael Emmerich von der Universität Leiden in den Niederlanden und seinem Betreuer Boris Naujoks von der TH Köln schrieb Patrick Echtenbruck eine Publikation zum Thema "A Multiobjective Approach to Classification in Drug Discovery". Eingereicht wurde die Publikation bei der "16th IEEE International Conference on Computational Intelligence in Bioinformatics and Computational Biology". Das Paper wurde akzeptiert und für den Best Paper Award nominiert. Die Veröffentlichung findet nach der Präsentation auf der Konferenz statt.
Abstract:
Classification based on machine learning algorithms is a widely used technique in contemporary in silico methods for drug discovery. However, typically the performance of the classification tool is evaluated based on a scalar performance score and essential information, such as the balance between false positive rates (FPRs) and false negative rates (FNRs) is not directly assessed. Moreover, there might be a large number of molecular features that are not relevant for the classification task and merely slow down the computations or add noise to the learning process. In this paper we adopt an approach that previously was used for the classification of text messages (spam/no-spam) to the classification of drug compounds (active/inactive). By considering the minimization of the classification costs (FPR, FNR) and the minimization of the number of features as separate optimization tasks, we demonstrate that it is possible to develop a more informative and versatile tool for drug discovery. We show, how to derive and evaluate 2-D and 3-D Pareto fronts for the classification of small compounds in active and non-active (similar studies could be conducted for toxic/non-toxic classification, and on other chemically relevant properties). We demonstrate the applicability of the method on a small data set for bio-activity prediction of ligands.
Juni 2019