Jörg Stork als hervorragender Doktorand ausgezeichnet
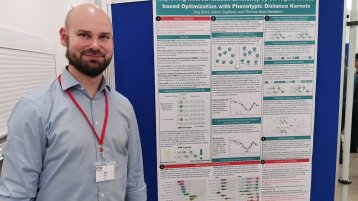
Jörg Stork, Doktorand im Institut IDEA, wurde im Rahmen der EvoStar Konferenz 2019 von der SPECIES Society als "Outstanding Student" ausgezeichnet. In diesem Jahr fand die EvoStar Konferenz vom 24. - 26. April in Leipzig statt. Die Konferenz zählt zu den führenden Veranstaltungen im Bereich der natur-inspirierten Optimierung.
Der "EvoStar Outstanding Students 2019" Preis wird an Studentinnen und Studenten verliehen, die die Zukunft der Forschungsgemeinschaft repräsentieren.
In EvoStar 2019 we wanted to recognise the good work of our students, who represent the future of our community.
Jörg Stork, der als wissenschaftlicher Mitarbeiter und Doktorand im Team von Prof. T. Bartz-Beielstein tätig ist, führt seine Promotion in Kooperation mit der Vrije Universiteit Amsterdam durch. Dort wird er von Prof. Gusz Eiben betreut, einem der weltweit führenden Forscher im Bereich evolutionärer Algorithmen und Robotik. Zusätzlich zu seiner Dissertation arbeitet Jörg Stork im Forschungsprojekt KOARCH. Im Projekt KOARCH werden Methoden des maschinellen Lernens, Condition-Monitoring- und Diagnose-Algorithmen und Optimierungsverfahren untersucht und implementiert.
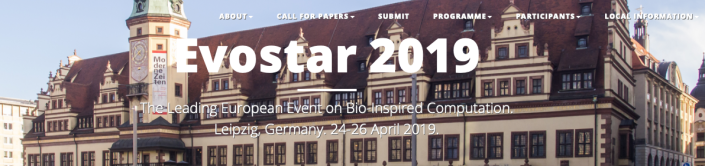
Jörg Stork präsentierte sowohl in einem Vortrag als auch als Poster seine wissenschaftliche Arbeit im Bereich der Anwendungen evolutionärer Algorithmen mit dem Titel “Improving NeuroEvolution Efficiency by Surrogate Model-based Optimization with Phenotypic Distance Kernels”.
Abstract:
"In NeuroEvolution, the topologies of artificial neural networks are optimized with evolutionary algorithms to solve tasks in data regression, data classification, or reinforcement learning. One downside of NeuroEvolution is the large amount of necessary fitness evaluations, which might render it inefficient for tasks with expensive evaluations, such as real-time learning. For these expensive optimization tasks, surrogate model-based optimization is frequently applied as it features a good evaluation efficiency. While a combination of both procedures appears as a valuable solution, the definition of adequate distance measures for the surrogate modeling process is difficult. In this study, we will extend cartesian genetic programming of artificial neural networks by the use of surrogate model-based optimization. We propose different distance measures and test our algorithm on a replicable benchmark task. The results indicate that we can significantly increase the evaluation efficiency and that a phenotypic distance, which is based on the behavior of the associated neural networks, is most promising."
Referenz:
Stork, J., Zaefferer, M., and Bartz-Beielstein, T. Improving neuroevolution efficiency by surrogate model-based optimization with phenotypic distance kernels. In Applications of Evolutionary Computation - 22nd International Conference, EvoApplications 2019, Held as Part of EvoStar 2019, Leipzig, Germany, April 24-26, 2019, Proceedings (2019), P. Kaufmann and P. A. Castillo, Eds., vol. 11454 of Lecture Notes in Computer Science, Springer, pp. 504–519.
Mai 2019