Comparison of Parallel Surrogate-Assisted Optimization Approaches - article published
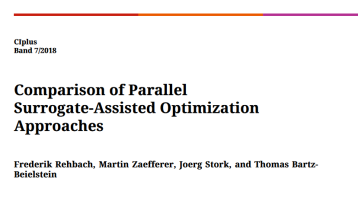
The article "Comparison of Parallel Surrogate-Assisted Optimization Approaches" has now been published on Cologne Open Science
The article was written by four members of the Institute for Data Science, Engineering, and Analytics (Frederik Rehbach, Martin Zaefferer, Joerg Stork, and Thomas Bartz- Beielstein). Its abstract reads as follows:
"The availability of several CPU cores on current computers enables parallelization and increases the computational power significantly. Optimization algorithms have to be adapted to exploit these highly parallelized systems and evaluate multiple candidate solutions in each iteration. This issue is especially challenging for expensive optimization problems, where surrogate models are employed to reduce the load of objective function evaluations. This paper compares different approaches for surrogate modelbased optimization in parallel environments. Additionally, an easy to use method, which was developed for an industrial project, is proposed. All described algorithms are tested with a variety of standard benchmark functions. Furthermore, they are applied to a real-world engineering problem, the electrostatic precipitator problem. Expensive computational fluid dynamics simulations are required to estimate the performance of the precipitator. The task is to optimize a gas-distribution system so that a desired velocity distribution is achieved for the gas flow throughout the precipitator. The vast amount of possible configurations leads to a complex discrete valued optimization problem. The experiments indicate that a hybrid approach works best, which proposes candidate solutions based on different surrogate model-based infill criteria and evolutionary operators."
The article is available electronically :
https://cos.bibl.th-koeln.de/frontdoor/index/index/docId/789
November 2018